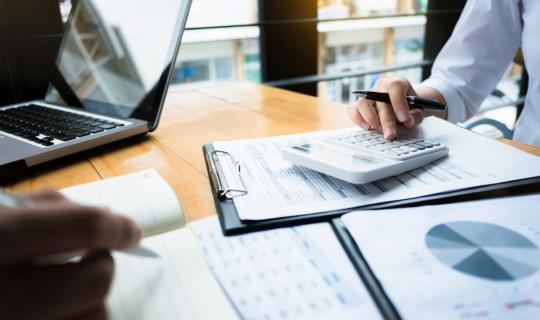
AI & E-Invoicing: Generix’s Solutions for the Future of Digital Invoicing
During the opening conference at the Solutions trade show, which focused on AI & dematerialization, the discussions were sparked with…
Rexel Italy boosts its intralogistics performance with Generix WMS View the press release
By nature, supply chain activities produce a high volume of data related to delivery rounds, storage of merchandise and MANQUE LE LIEN >> order preparation. With artificial intelligence, this information can now be put to use to optimize the logistics chain via predictive analysis.
In the field of distribution, machine learning algorithms now offer unrivaled prediction features when estimating changes in activity. They make it possible to evaluate the combined influence of a plethora of internal and external demand forces, while also considering the particularities of each sector.
Machine learning operates on data that distribution partners transmit to sellers. More specifically, it is used to detect criteria that might not otherwise have been expected to have an impact on demand. Armed with this knowledge, the distributor can handle inventory, merchandising and the movement of goods with finesse, in order to market the right products at just the right moment and, more importantly, in sufficient quantity and via the appropriate channels.
On the warehouse end, order preparation is subject to major load peaks. Team managers often need to limit internal resources to optimize costs and call on temporary workers when consumption is particularly high. To help novice operators make the right decisions and bring them up to a productive speed more quickly, managers have the option of using artificial intelligence and machine learning solutions.
Take the example of an operator whose job is to load boxes onto pallets. By analyzing package dimensions, the algorithm can choose the best way to position them on the pallet (laying down, on the side, vertically, etc.) to optimize loading and reduce the number of pallets to be shipped.
At the end of the chain, these decisions have a considerable impact on transport costs and customer satisfaction, as recipients can receive their package without waiting for the next truck to make rounds. Also, optimizing the merchandise pickup circuit lets order preparers prevent unnecessary trips and become quicker and more efficient.
As regards planning, AI can help warehouse managers predict the number of operators necessary to absorb peaks in activity. By analyzing one period in particular against demand predictions, they can precisely calculate the additional resources necessary and increase the performance of a logistics site.
Using intelligent sensors, companies on the supply chain have at their disposal new means to prolong the lifespan of their transport vehicles and warehouse management equipment. Data emitted by IoT devices make it possible to detect factors that influence machine lifespan and come up with new use models. With machine learning, it becomes easier to measure Overall Equipment Effectiveness (OEE), a key performance indicator for the supply chain.
To ensure end-to-end visibility for all operations on the supply chain, real-time surveillance is a must. Combined with IoT sensors and advanced machine learning algorithms, a digital platform implemented with data accessible in real-time can allow for full-fledged analysis.
To closely study behavior and come up with reliable prediction models, an artificial intelligence solution must have access to high volumes of information to analyze. This is a non-negotiable prerequisite for proper algorithm functioning. Three weeks of history is not sufficient to get a machine learning application up and running, as there would not be enough data to work with.
Storage of big data is a major challenge for companies. They need to:
In order for data to be usable in real-time, it is also necessary to evaluate data history and understand some information that has become obsolete. If a reference no longer exists, it may be necessary to consider the behavior of its family rather than seek to work with the reference itself.
To be sure AI engines remain fully operational and continue to benefit from their predictive features, supply chain companies must immediately begin storing their data. To do so, they must quickly construct a sufficient database for future operations.
During the opening conference at the Solutions trade show, which focused on AI & dematerialization, the discussions were sparked with…
With the CSRD, the European directive on corporate sustainability reporting, imposing a new reporting timeline, large companies and SMEs (Small…
Currently, 63 PDPs are conditionally registered (+3 companies have submitted two applications), 7 are in the registration process, and about…
Work with our team to build your ideal supply chain software stack and tailor it to your unique business needs.